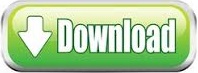

Where is the predicted of expected systolic blood pressure. The mean BMI in the sample was 28.2 with a standard deviation of 5.3.Ī simple linear regression analysis reveals the following: A total of n=3,539 participants attended the exam, and their mean systolic blood pressure was 127.3 with a standard deviation of 19.0. Suppose we want to assess the association between BMI and systolic blood pressure using data collected in the seventh examination of the Framingham Offspring Study. The Association Between BMI and Systolic Blood Pressure The test of significance of the regression coefficient associated with the risk factor can be used to assess whether the association between the risk factor is statistically significant after accounting for one or more confounding variables. Once a variable is identified as a confounder, we can then use multiple linear regression analysis to estimate the association between the risk factor and the outcome adjusting for that confounder. As a rule of thumb, if the regression coefficient from the simple linear regression model changes by more than 10%, then X 2 is said to be a confounder. In this case, we compare b 1 from the simple linear regression model to b 1 from the multiple linear regression model. As noted earlier, some investigators assess confounding by assessing how much the regression coefficient associated with the risk factor (i.e., the measure of association) changes after adjusting for the potential confounder. In the multiple linear regression equation, b 1 is the estimated regression coefficient that quantifies the association between the risk factor X 1 and the outcome, adjusted for X 2 (b 2 is the estimated regression coefficient that quantifies the association between the potential confounder and the outcome). If we now want to assess whether a third variable (e.g., age) is a confounder, we can denote the potential confounder X 2, and then estimate a multiple linear regression equation as follows: Where b 1 is the estimated regression coefficient that quantifies the association between the risk factor and the outcome. We can estimate a simple linear regression equation relating the risk factor (the independent variable) to the dependent variable as follows: Suppose we have a risk factor or an exposure variable, which we denote X 1 (e.g., X 1=obesity or X 1=treatment), and an outcome or dependent variable which we denote Y. Identifying & Controlling for Confounding With Multiple Linear RegressionĪs suggested on the previous page, multiple regression analysis can be used to assess whether confounding exists, and, since it allows us to estimate the association between a given independent variable and the outcome holding all other variables constant, multiple linear regression also provides a way of adjusting for (or accounting for) potentially confounding variables that have been included in the model. Again, statistical tests can be performed to assess whether each regression coefficient is significantly different from zero. In the multiple regression situation, b 1, for example, is the change in Y relative to a one unit change in X 1, holding all other independent variables constant (i.e., when the remaining independent variables are held at the same value or are fixed). Each regression coefficient represents the change in Y relative to a one unit change in the respective independent variable. Where is the predicted or expected value of the dependent variable, X 1 through X p are p distinct independent or predictor variables, b 0 is the value of Y when all of the independent variables (X 1 through X p) are equal to zero, and b 1 through b p are the estimated regression coefficients. The multiple linear regression equation is as follows:
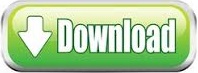